New Model Uses Satellite Imagery, Machine Learning to Map Flooding in Urban Environments
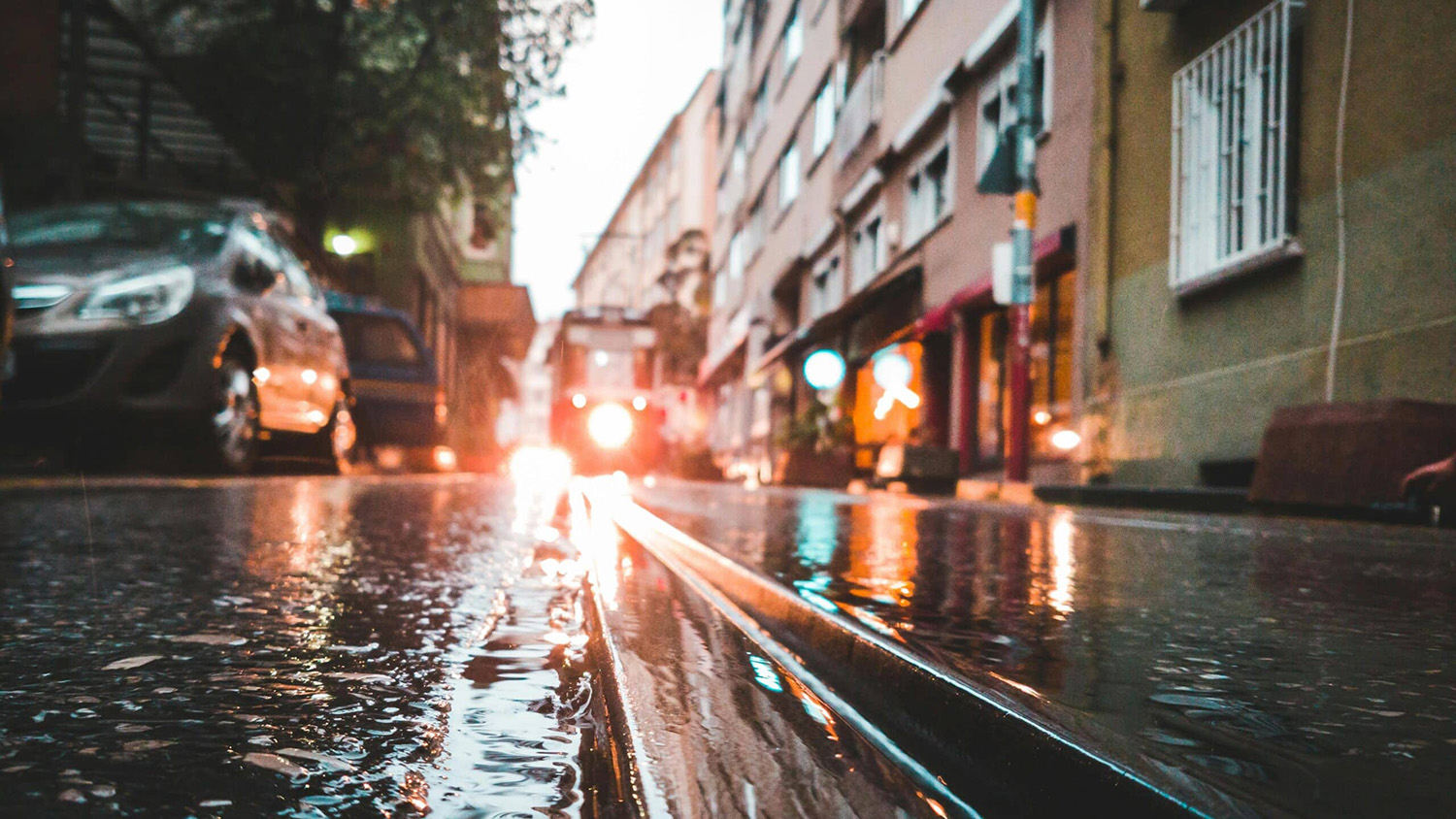
As climate change causes storms to intensify, new tools are needed to map where flooding occurs in under-studied areas. NC State researchers have developed a model that could help.
Rebecca Composto, a graduate student in the Center for Geospatial Analytics, used machine learning and open-source satellite imagery to create a model capable of generating maps that predict urban area flooding, which traditionally have not been accessible to urban planners.
Urban areas present unique challenges in collecting satellite data and tracking the flow of water, according to Composto. The maps could help identify potentially flood-prone areas in urban settings, helping officials make better-informed choices about where to allocate flood resiliency and prevention resources.
“One of the first issues is building shadows. Taller buildings create more shadows, which means that the satellite imagery appears darker and carries less information,” Composto said. “Urban areas also have more complex hydrology, as the existence of so many drainage systems along with concrete surfaces that don’t soak up water means that it’s harder to predict where water accumulates.”
Flooding in urban areas also tends to both start and end quickly, sometimes too quickly for satellites to gather enough usable data. To address this, Composto used satellite data from Hurricane Ida, which caused significant flooding and damage in the northeastern U.S. in 2021. Flooding from Ida lasted longer than usual in areas like Philadelphia, and this combined with a sudden break in cloud cover gave Composto just the kind of satellite data she needed.
The next step was to train a machine-learning model to recognize and map flooding. To create sufficient training data for the model to recognize urban flooding, Composto spent months hand-drawing polygons onto her satellite imagery to help the program understand what it was looking at. These polygons helped the machine-learning algorithm “see” the characteristics of satellite imagery most aligned with flooding and then map the flooding.
Composto then compared the resulting map, known as a flood extent, to Federal Emergency Management Agency flood zones. These zones designate areas depending on how likely they are to flood – for example, a “500-year” flood zone has an approximately 1-in-500 chance of flooding each year. Other areas are deemed “minimal flood hazard,” which places them outside the limit of the 500-year flood designation.
The model found that more flooding was occurring in these minimal hazard areas than in the 500-year zones. Composto said that this was likely due to the much larger size of the minimal hazard zones – despite having more flooding by volume, the risk of flooding in these areas was still proportionally smaller than in the 500-year zones.
Further research might focus on simplifying the model for ease of use. Composto plans to integrate a new map displaying flood depth and make her code open source to make it easier to share with emergency-response leaders.
- Categories: