Strengthening Food Security with Better Flood Management
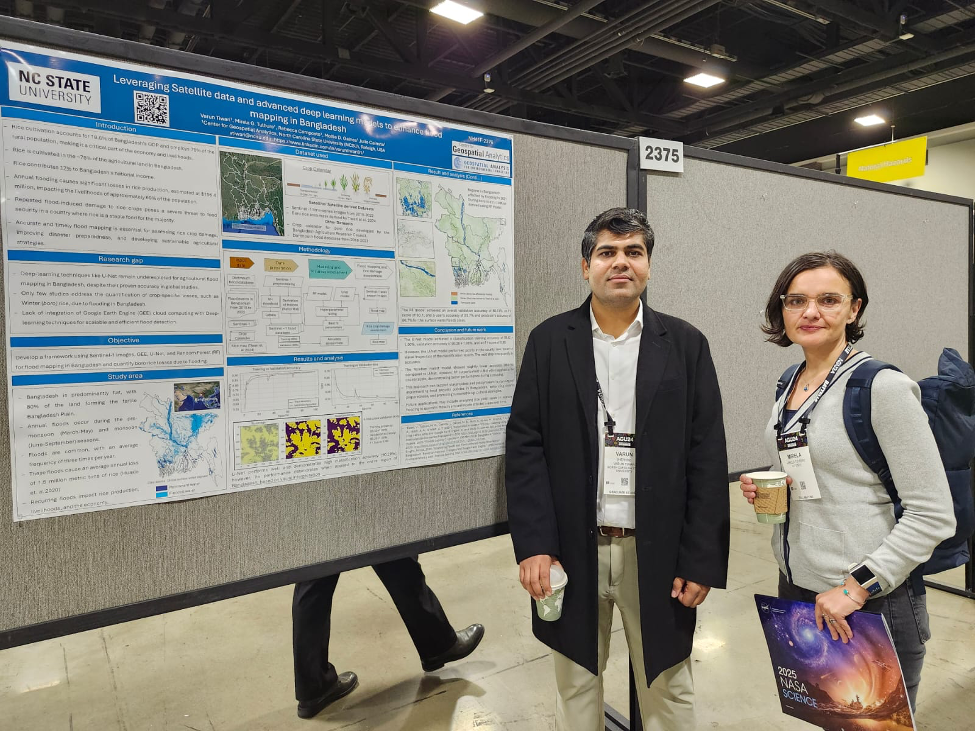
Editor’s note: Each semester, students in the Geospatial Analytics Ph.D. program can apply for a Geospatial Analytics Travel Award that supports research travel or presentations at conferences. The following is a guest post by travel award winner Varun Tiwari as part of the Student Travel series.
Importance of rice in Bangladesh and its vulnerability to flooding
In Bangladesh, rice isn’t just food — it’s life. For 165 million people, rice is the main meal on their plates. For farmers, it’s their biggest source of income. It also plays a huge role in the country’s economy, making up 70% of agricultural GDP and about one-sixth of the country’s total income.
But climate change is making things hard. Unpredictable weather and stronger floods are causing big problems, especially for winter rice, called Boro. Bangladesh is already one of the most climate-affected countries in the world, and these changes are hitting farmers and rice production hard.
Floods are damaging rice crops, lowering yields, and making life tougher for farmers. To tackle this, we need accurate information about floods—like when and where they happen and how much damage they cause. With better data, Bangladesh can reduce crop losses, improve food security, and support farmers more effectively.
Role of earth observation in mapping floods
Thanks to modern technology, satellites can help us see floods like never before. Using Earth observation data, we can map floods and figure out how they affect rice fields. Two main types of satellite data are used: optical and Synthetic Aperture Radar (SAR).
Optical sensors, like the ones on Sentinel-2 and Landsat satellites, take clear pictures of the Earth in visible and infrared light. These sensors are great for spotting water, land changes, and vegetation. But there’s a problem—optical sensors can’t see through clouds. During monsoon season in Bangladesh, when it’s cloudy all the time, these sensors can’t do their job well.
That’s where SAR sensors step in. SAR satellites, like Sentinel-1, use microwaves that can see through clouds and even work at night. This makes them perfect for mapping floods in places like Bangladesh, where heavy rains and clouds are common. With SAR, we can create detailed flood maps that show where and when floods happen. These maps help us understand the damage to rice fields and plan better ways to protect crops.
Do we need a deep learning model to map floods in data-scarce regions such as Bangladesh?
When it comes to flood mapping, artificial intelligence (AI) techniques like machine learning (ML) and deep learning (DL) have been game-changers. Both are data-driven, but there’s a big catch: DL needs a lot more data and computing power to deliver accurate results. Models like U-Net, for example, are incredibly powerful but come with hefty demands for resources, which can be both time-consuming and carbon-intensive. Sure, DL often wins on accuracy, but it begs the question: Do we really need deep learning for flood mapping in data-scarce regions like Bangladesh? Or is it like using a machine gun to kill a mosquito when something simpler—like mosquito repellent—might do the job just fine?
This year, I had the opportunity to attend and present my work in person at the American Geophysical Union (AGU) Fall Meeting, held in Washington, D.C., from December 9 to 13, 2024. I was thrilled and honored to receive a Travel Award from the Center for Geospatial Analytics, which made this experience possible. During the event, I presented the third chapter of my Ph.D. dissertation, which focuses on using satellite data and deep learning techniques for flood mapping in Bangladesh. The meeting provided an excellent platform to share my research, exchange ideas, and connect with experts in the field.
In my study, I compared two methods: the advanced U-Net deep learning model and the simpler random forest machine learning model. Both models were trained using Sentinel-1 SAR data during the rice-growing season in Bangladesh.
U-Net performed impressively in small-scale tests, achieving 93% accuracy. However, when I tried to use it for larger, county-level flood maps, its accuracy dropped significantly.
On the other hand, the random forest model, while slightly less accurate (91% overall accuracy—only 2% lower than U-Net), handled large-scale mapping much better. It needed less training data, worked well with noisy inputs, and didn’t require supercomputers to run. In a country like Bangladesh, where data and resources are limited, random forest proved to be the better choice.
The takeaway? Deep learning might sound exciting and innovative, but it’s not always the best option. Sometimes, a simpler and more efficient method—like random forest—is not just good enough; it’s actually better.
Rice crop affected by flooding in Bangladesh
To understand how flooding impacts rice crops, we overlaid the flood map with the rice map from 2021, developed as part of Chapter 1 of my Ph.D. dissertation. The results revealed that 4.2% of the total Boro rice area is affected by flooding, leading to expected declines in yields and productivity.
This method can be applied in the future for different years to pinpoint areas most vulnerable to flooding. Such insights are invaluable for government decision-making. They can help guide changes in crop patterns, adjust rice cropping cycles, introduce flood-tolerant rice varieties, explore alternative crops, and develop long-term strategies to ensure food security in flood-prone regions.
- Categories: