Ph.D. in Geospatial Analytics
Our innovative Ph.D. program brings together researchers from across NC State University to train a new generation of interdisciplinary data scientists skilled in developing novel understanding of spatial phenomena and in applying new knowledge to grand challenges.
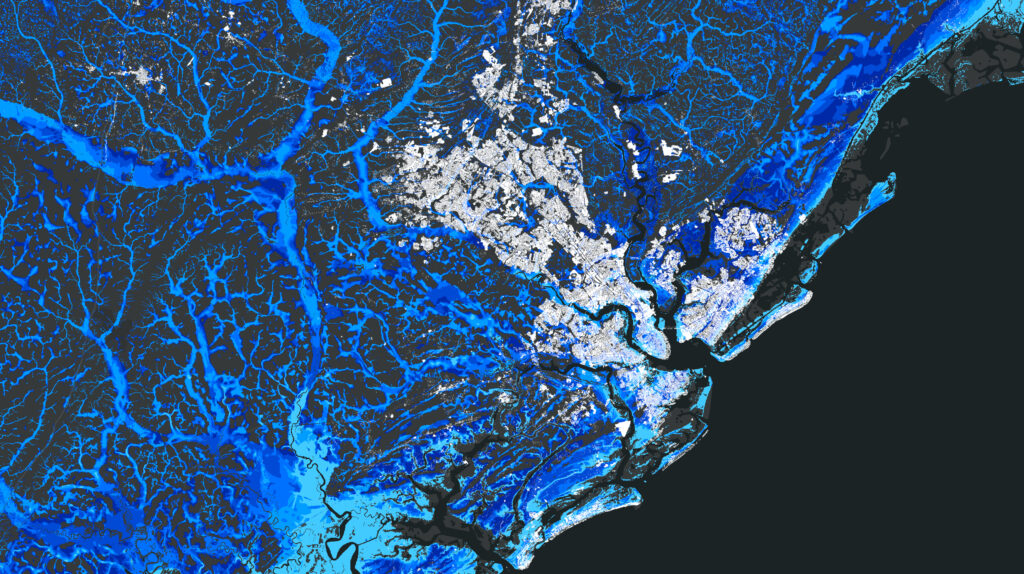
This one-of-a-kind degree focuses on integrative thinking and experiential learning:
- Collaborative, cross-disciplinary teamwork unites students and faculty from many research fields
- Guaranteed funding for four years includes a competitive minimum stipend of $30,000, health insurance, and tuition
- Professional seminar supports student success through training in science communication, proposal writing and geospatial data ethics
- Travel funding is available for students to attend scientific conferences
- Program values include prioritizing student mental health and work/life balance, open data, environmental and social justice, and a commitment to collaboration, community and equity
If your research goals intersect geospatial problem-solving from any number of fields, you will find your fit here. Our Faculty Fellows advise students interested in a range of disciplines––from design, to social and behavioral sciences, natural resources and the environment, computer science, engineering and more––and approach their work in a range of geospatial research areas. Students with strong backgrounds in quantitative methods in geography, data science, remote sensing and earth sciences are strongly encouraged to apply. We are especially committed to increasing the representation of students that have been historically excluded from participation in U.S. higher education.
Find recent publications by our students and faculty through NC State’s Libraries Citation Index and learn more about the achievements of our students and alumni.
Apply for a Ph.D. in Geospatial Analytics
Ten fully funded Ph.D. graduate assistantships with $30,000 salary, benefits, and tuition waiver are available for Fall 2024 through the Center for Geospatial Analytics.
Applications for Fall 2024 admissions are now open. The application deadline is February 1, 2024 – all recommendations and test scores must be received by this date.
There are several opportunities for students to receive a stipend above the base rate of $30,000. These fellowships do not require an additional application:
- Goodnight Doctoral Fellowship. One to two incoming students with a track record of exceptional achievement in the sciences will earn an additional $10,000 per year + all student fees waived for four years
- University Graduate Fellowship. Five incoming students will receive an additional $4,000 in their first year
- Diversity Enhancement Fellowship. Two incoming students will receive an additional $2,000 in their first year
- Mansour Doctoral Fellowship. One incoming international student will be nominated to receive an additional $10,000 in their first year
Admission Requirements
Our most competitive applicants will have
- Significant quantitative research experience outside of the classroom, beyond basic data collection or data entry
- Computational/quantitative background, including a combination of the following coursework or demonstrated skills: statistics, advanced mathematics, quantitative research methods, R, Python
- Prior coursework, background and/or research interests in the area of geospatial analytics
- For international applicants: IBT TOEFL score ≥ 80 overall (18 in each section), IELTS score ≥ 6.5 on each section, Duolingo English ≥ 110. Scores are not required for citizens of these countries or who have completed at least one year of full time study at U.S. college or university
Supporting Documents
- Official NC State Graduate School application.
- Unofficial transcripts from all colleges/universities attended (official transcripts are only required if admitted to the program).
- A personal statement, not to exceed 2 pages. We encourage you to consider the following:
- Your academic and career goals as well as your motivation in pursuing a Ph.D.
- Research experiences and background/skills that would make you a successful Ph.D. student in geospatial analytics
- Relevant research interests
- Your specific interest in the Ph.D. in Geospatial Analytics at NC State
- 3 letters of recommendation. Submit the names and contact information for your recommenders through the online application, and they will receive an email with instructions for submitting their letters online. Please select recommenders who can speak to your academic and/or research potential.
- Curriculum vitae/resume.
- Optional GRE scores. Taking the GRE is strongly recommended for international students who have not previously studied in the U.S.
If you have questions about the application process, please contact Rachel Kasten, Graduate Services Coordinator (rachelkasten@ncsu.edu, 919-515-2800). Please note that there is a required application fee of $75 for domestic applicants and $85 for international applicants. McNair Scholars will have the application fee waived. This fee cannot be waived or reduced for international students.
More information for prospective international students can be found here.
Degree Requirements
The Ph.D. program consists of
- 72 credit hours beyond the Bachelor’s degree. The core required courses comprise 18 credit hours. The remaining 54 credit hours are devoted to an individually tailored selection of electives and research.
- an off-campus professional experience. By the beginning of their third year in the program, students participate in an experiential learning activity within government (local, state, federal), industry, private and academic research institutions, or other organizations in the geospatial arena. Students consult with their advisors to identify specific opportunities that will enhance their doctoral program.
- a comprehensive exam. Students will complete both written and oral exams by the end of their fifth semester in order to be admitted to candidacy.
- a written dissertation and final dissertation oral defense required to complete the degree.
Core Curriculum
The core curriculum includes the following courses; click course names to view descriptions. Students are required to take GIS 710 and any three additional core courses, as well as six elective credits:
GIS 710: Geospatial Analytics for Grand Challenges
Students examine why sustainable solutions to grand societal challenges need geospatial analytics. Emphasis is placed on the roles that location, spatial interaction and multi-scale processes play in scientific discovery and communication. Discussion of seminal and leading-edge approaches to problem-solving is motivated by grand challenges such as controlling the spread of emerging infectious disease, providing access to clean water and creating smart and connected cities. Students also engage in several written and oral presentation activities focused on data science communication skills and professionalization.
GIS 711: Geospatial Data Management
Applied experience in the architecture of geospatial data management, including open source options. The course introduces students to: (i) spatial and temporal data types (OGC specification, GPS and accelerometer matching), (ii) spatial predicates, (iii) spatial indices and (iv) spatial query processing. In addition, students will be exposed to modern spatial data management systems like NoSQL and graph databases, and data integration principles including protected health information (PHI/HIPAA).
GIS 712: Environmental Earth Observation and Remote Sensing
Advanced understanding of physical principles of remote sensing, image processing and applications from earth observations. Awareness of tradeoffs between earth observing sensors, platforms and analysis techniques will help prepare the students to critically assess remote sensing products and devise solutions to environmental problems. Students will be able to communicate the complexities of image analysis and will be better prepared to integrate earth observations into their areas of expertise. Topics include electromagnetic energy and radiative transfer; US and international orbital and suborbital data acquisition platforms; passive and active imaging and scanning sensors; spatial, spectral, radiometric, and temporal resolutions; geometric corrections and radiometric calibrations; preprocessing of digital remotely sensed data; advanced image analysis including enhancement, enhancement, classification, geophysical variable retrieval, error and sensitivity analysis; data fusion; data assimilation; and integration of remotely sensed data with other data types in a geospatial modeling context.
GIS 713: Geospatial Data Mining and Analysis
Spatial data mining is the process of discovering interesting and previously unknown, but potentially useful, patterns from spatial and spatiotemporal data. However, explosive growth in the spatial and spatiotemporal data (~70% of all digital data), and the emergence of geosocial media and location sensing technologies has transformed the field in recent years. This course reviews the current state of the art in spatial, temporal and spatiotemporal data mining and looks at real-world applications ranging from geosocial networks to climate change impacts. Course introduces various spatial and temporal pattern families and teaches how to incorporate spatial relationships and constraints into data mining approaches like clustering, classification, anomalies and colocations.
GIS 714: Geospatial Computation and Simulation
Methods, algorithms and tools for geospatial modeling and predicting spatio-temporal dimensions of environmental systems. The course covers the physical, biological, and social processes that drive dynamics of landscape change. Deterministic, stochastic, and multi-agent simulations are explained, with emphasis on coupling empirical and process based models, techniques for model calibration and validation and sensitivity analysis. Applications to real-world problems are explored, such as modeling multi-scale flow and mass transport, spread of wildfire, biological invasions and urbanization.
GIS 715: Geovisualization
Principles of visualization design and scripting for geospatial visualization. This course provides a systematic framework of visualization design principles based on the human visual system and explores open-source geospatial data visualization tools. Topics include challenges and techniques for visualizing large multivariate dataset, spatio-temporal data and landscape changes over time. Students have the opportunity to work with broad range of visualization technologies, including frontiers in immersive visualization, tangible interaction with geospatial data and eye tracking.
Frequently Asked Questions
Below are some of the most frequently asked questions we have received about the Ph.D. program in Geospatial Analytics. If your questions are still not answered here, please feel free to contact us through the form below.
Can the program be completed online or part-time?
No, the Ph.D. in Geospatial Analytics is a traditional full-time on-campus program.
I am currently in a master’s degree program and will complete my degree in the spring. Can I still apply now to start the Ph.D. program in the fall?
Yes. We accept unofficial transcripts with your application. Official transcripts will be requested if you are admitted to the program.
Do I need to have been a geography major to apply?
No, we welcome applications from students with strong computational skills from diverse backgrounds, including computer science, data science, environmental science, ecology, engineering, and more.
Do I need a master’s degree to apply?
No, students may enroll without a master’s degree. Successful applicants, however, will have had previous academic research experience.
Do you offer application fee waivers?
Application fee waivers are offered only for domestic students who have participated in specific research programs (i.e. McNair Scholars).
Is financial assistance available?
Incoming doctoral students receive a tuition waiver, health insurance benefits, and a $30,000 stipend.
Do I need to secure an advisor before applying?
While you are encouraged to connect with faculty who share your interests prior to applying (the application will ask you to name a preferred advisor), students can be admitted on program funding without a specific advisor/position.
What kinds of projects might I work on?
Students in the Geospatial Analytics doctoral program work on a diverse range of data science frontiers intersecting multiple disciplines, with funding available from the Ph.D. program as well as from external grants secured by faculty. Assistantships are each fully funded for four years. Below are a sample of the opportunities that were available in previous years. For more details about each opportunity, and to learn about past projects, visit our Graduate Assistantships page.
- Landscape Connectivity Dynamics in Surface Water Networks — Join the Geospatial Analysis for Environmental Change Lab to investigate climate and land-use change effects on landscape connectivity dynamics.
- Seasonality from Space — Join the Spatial Ecosystem Analytics Lab on a NASA-funded project investigating satellite data fusion and time series analysis.
- Winter Weather — Join the Environment Analytics group to study the complex interactions within snow storms and wintery mix storms.
- Modeling Forest and Water Resources under Changing Conditions — Join the Watershed Ecology lab group and combine various data sources to create projections of future landscape conditions.
- Modeling Agricultural and Water Resource Dynamics — Join the Biosystems Analytics Lab to study the effects of global and local change on fresh and estuarine water quality, land-sea connectivity and agroecosystem productivity.
- Surface Water Dynamics from Space — Join the Geospatial Analysis for Environmental Change Lab to investigate hydroclimatic drivers of surface water extent dynamics and advance quantification of water extent and volume.
- Remote Sensing Forest Gap Dynamics — Join the Applied Remote Sensing and Analysis lab group to examine the role and influence of forest gaps in relation to localized large-scale disturbances.
Funding is available for additional projects, and in all cases students are encouraged to develop research questions and methods that suit their interests and career goals.
Questions?
We’re here to help! Contact us for more information about the Ph.D. in Geospatial Analytics.
Explore Opportunities
Our graduate assistantships are fully funded with a yearly stipend, tuition support, and benefits. Learn more about opportunities at NC State and the Research Triangle to enrich your graduate experience.