Exploring Scenario-Based Surface Water Area Projections at AGU
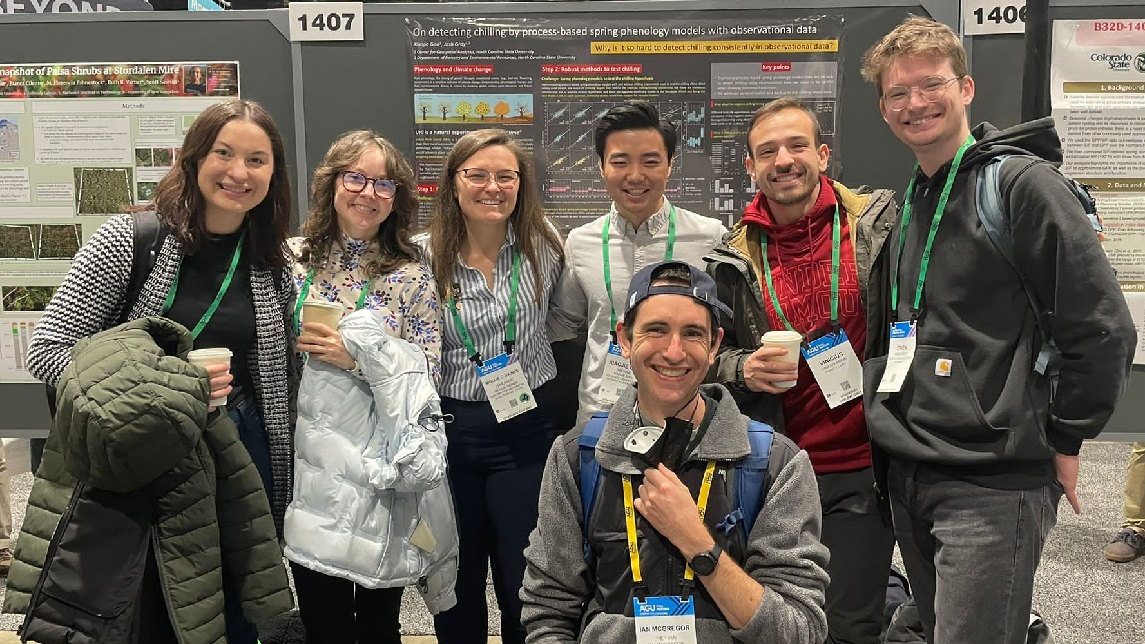
Editor’s note: Each semester, students in the Geospatial Analytics Ph.D. program can apply for a Geospatial Analytics Travel Award that supports research travel or presentations at conferences. The following is a guest post by travel award winner Mollie Gaines as part of the Student Travel series.
Attending the American Geophysical Union (AGU) Fall Meeting is becoming one of my favorite academic endeavors. In December of 2022, and with the help of the Travel Award from the Center for Geospatial Analytics, I presented my research at my second AGU Fall Meeting. Throughout the week, I reconnected with former colleagues, swapped ideas with potential collaborators and met scientists from a range of Earth and space related disciplines. Last, but not least, I was able to explore the sights and tastes of Chicago—from seeing The Bean (Cloud Gate) to eating Lou Malnati’s deep dish pizza to drinking a hot chocolate at Wrigley Field in the snow.
The AGU Fall Meeting is an enormous scientific conference, with over 25,000 attendees and thousands of sessions. I presented my research at a hydrological session titled “Advances in Quantifying Impacts and Extents of Land-Use/Land-Cover Change on Hydrology and Climate Change.”
My research is specifically focused on surface water dynamics in the southeastern United States. This region is experiencing more land-cover/land-use change than any other region in the country, with much of this change converting forested areas to cropland or urban areas. Building on research I’d previously published (Gaines et al. 2022), the work I presented focused on using machine learning to project surface water area in the Southeast under different climate and land cover change scenarios.
Water stress is a global concern as a changing climate leads to variations in weather patterns. This issue is compounded by agricultural and urban areas using water-intensive practices. Urban areas already use close to their water resource limit but continue to expand across the Southeast. Understanding spatial and temporal drivers of surface water dynamics—e.g., temperature, precipitation and land cover change—is key to more effectively managing resources and limiting the effects of water stress. So far, these drivers have been assessed mainly using local-scale hydrological models, with many not accounting for human drivers such as land-use and land-cover change. We produced scenario-based surface water area projections to 2100 that combined land-cover and climate projections under different development and emissions scenarios for the southeastern United States.
Scenario-based projection models that include a worst-case scenario are useful to water resource planners to robustly prepare for future water stress. In our preliminary results, we found that our most extreme scenario—high greenhouse gas emissions paired with continued population growth and slower technological development—yielded the most extreme changes in surface water area at the watershed scale. Our next steps are to scale our model up to 10,000 simulations per scenario and to compare our machine learning results to a process-based model similarly focused on changes to above-ground water resources due to land-cover and climate change.
After my presentation, I connected with another graduate student conducting similar research using a process-based hydrological model to assess the impact of climate and land-cover change on water resources. We ended up discussing different aspects of our research for nearly two hours, and exchanged information to continue collaborating in the future. In my experience, such organic meetings with potential collaborators are unique to scientific conferences, and I am thankful to the CGA for supporting my attendance this year.
Acknowledgements:I would like to thank Dr. Mirela G. Tulbure, Dr. Vinicius Perin and Varun Tiwari for their work on this project. I would also like to thank the NASA SCoPE Affiliate program for awarding me additional funding to travel to and present at the 2022 AGU Fall Meeting.
- Categories: