Efficiently Monitoring Small Farms from Space
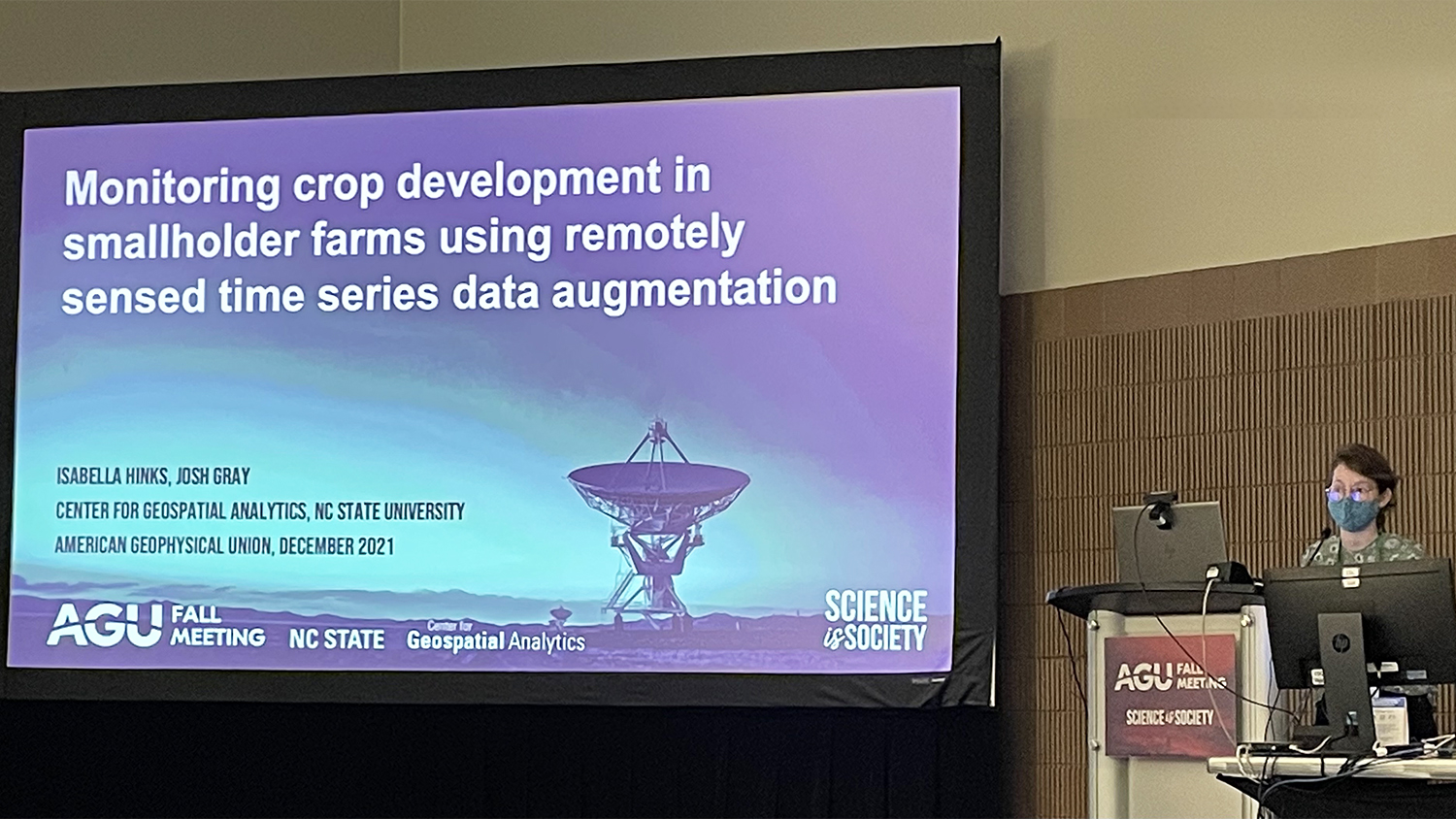
Editor’s note: Each semester, students in the Geospatial Analytics Ph.D. program can apply for a Geospatial Analytics Travel Award that supports research travel or presentations at conferences. The following is a guest post by travel award winner Izzi Hinks as part of the Student Travel series.
Smallholder farms, less than 5 acres in size, play an integral role in global efforts to achieve food security and foster sustainable agriculture. Despite only comprising about 12% of all agricultural land on Earth, they provide over 70% of food calories for people in Asia and sub-Saharan Africa (Fanzo 2017).
Unfortunately, many smallholder farming communities experience widespread food insecurity due to the compounding effects of climatic threats, rapidly growing populations and limited capital. Smallholder farmers can use accessible adaptations to increase their food production, but the extent to which these practices have already been adopted is largely unknown. Without this knowledge, it is impossible to determine how smallholder farms could further adapt.
I use time series of multi-source satellite imagery to monitor smallholder crop development across space and time, to ultimately quantify their adaptive potential. This will help determine how to make sustainable changes to feed a growing global population amid increasingly severe and frequent climate threats.
Remote sensing scientists can monitor changes in large agricultural fields using free imagery from public satellite programs –– and have been able to for decades. Contrastingly, despite recent advancements in the abundance and resolutions of public satellite data, we still struggle to monitor changes in smallholder fields using only public satellite imagery. The public satellites either offer imagery where the spatial resolutions are too coarse –– about 25 smallholder fields can fit in just one of MODIS’ 500 x 500-m pixels –– or the observations are too infrequent –– in the best scenario (without any pixels obstructed by clouds), Sentinel-2 and Landsat-8 satellites offer images every 5 and 16 days, respectively.
We can use commercial satellite imagery with higher spatial resolutions and more frequent observations, but the data quickly become financially and computationally costly. So, rather than using all available commercial satellite imagery, I hypothesized that we could augment time series of public satellite imagery with minimal amounts of commercial satellite images to monitor smallholders’ crop development. But this raised some questions: how do we know which images to buy, and how much commercial satellite imagery do we need?
I developed and compared several augmentation approaches to explore how minimal amounts of well-selected commercial imagery, identified beforehand, can be used to augment publicly available time series. Thanks to the Center for Geospatial Analytics (CGA) Fall 2021 Travel Award, I attended the American Geophysical Union (AGU) 2021 Fall Meeting in New Orleans, Louisiana, where I gave an oral presentation of this work and connected with the AGU community in person for the first time.
At AGU, I shared a use case in which we extracted crop growth stages of the 2017–2018 winter wheat season for 213 smallholder fields in Bihar, India. By augmenting time series of public Landsat 8 and Sentinel-2 imagery with minimal amounts of contextually useful commercial PlanetScope data, we reduced our use of expensive commercial satellite imagery by 75%. With only 25% of the PlanetScope images, we extracted crop development metrics of the smallholder fields with as little uncertainty as we achieved when we included all available PlanetScope observations.
I look forward to sharing this augmentation algorithm and my methodology for public use upon publication. This approach to prioritize available commercial images can be generalized to other applications, and is thus a valuable tool that allows remote sensing scientists to maximize their financial and computational expenditures.
Acknowledgements
Thank you to CGA’s Josh Gray for advising this work, and session conveners Yun Yang (University of Maryland), Martha Anderson (US Department of Agriculture) and Zhe Zhu (University of Connecticut) for providing me with the opportunity to contribute to session B42C at AGU.