Observing Landslide Events from Multi-Satellite Sensors
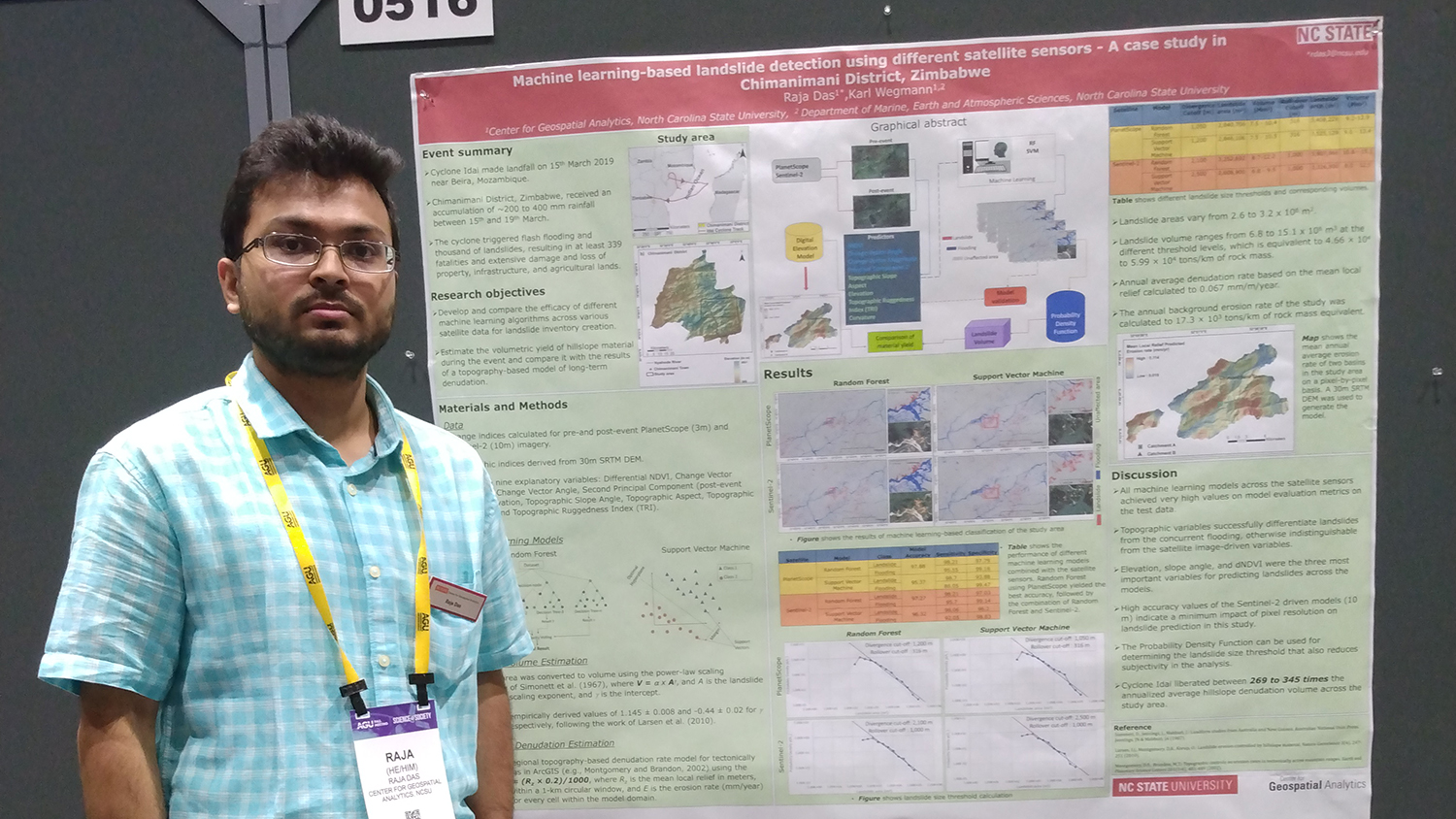
Editor’s note: Each semester, students in the Geospatial Analytics Ph.D. program can apply for a Geospatial Analytics Travel Award that supports research travel or presentations at conferences. The following is a guest post by travel award winner Raja Das as part of the Student Travel series.
Last year in December, I had the opportunity to present my work on the use of multi-satellite sensors in predicting landslides at the annual meeting of the American Geophysical Union (AGU), 2021. Thanks to the Center for Geospatial Analytics at NC State University for awarding me the grant to attend the conference.
Landslides are the most recurrent and devastating geological hazard in mountainous regions around the world. Globally, the yearly losses caused by landslides amount to billions of dollars of infrastructural and property damages and thousands of fatalities. These landslide events are most frequently triggered by heavy rainfall and less frequently by earthquakes. Researchers are concerned that the increasing frequency of extreme rainfall events as a result of global climate change will also cause an increase in landslide events in many parts of the world.
In recent years, the advancement of technology and emergence of high-resolution satellite imagery has enabled mapping landslides with greater detail and accuracy. The general understanding is that the finer the image resolution better the mapping accuracy. However, the procurement of high-resolution imagery involves a hefty cost, which on many occasions is the biggest impediment for many researchers.
In this study, I utilized satellite imagery and machine learning techniques to investigate the landslides triggered as an aftermath of Cyclone Idai. Cyclone Idai was the deadliest tropical cyclone in the southwestern part of the Indian Ocean; from March 15–19, 2019, the storm ripped through the south of Africa and triggered widespread landslides and concurrent flooding in major parts of Mozambique, Zimbabwe and Malawi. The event caused a loss of 1,300 lives and damage of about $2.2 billion.
The Chimanimani district of Zimbabwe was among the worst-hit places by Cyclone Idai and witnessed thousands of landslides along with concurrent flooding across the area. I worked on mapping the spatial extent of these landslides and flooding in the Chimanimani district using different satellite data and machine learning algorithms. Our results indicated that the cyclone alone generated a material yield of 15.3 to 19.6 million tons, which is 269 to 365 times that of the annual sediment yield under normal erosion rates.
Additionally, I comparatively evaluated the efficacy of various satellite imagery of different spatial resolutions (namely, PlanetScope [3m/pixel], RapidEye [5m/pixel] and Sentinel-2 [10m/pixel]) coupled with different machine learning algorithms (namely, Artificial Neural Network [ANN], Random Forest [RF] and Support Vector Machine [SVM]) in mapping landslides and flooding in the study area. Results revealed that although the high-resolution commercial PlanetScope imagery coupled with the RF model yielded the highest model accuracy, the freely available Sentinel-2 imagery coupled with the RF model achieved comparable results, with a loss of only 0.61% accuracy. This finding is particularly important for promoting the use of freely available data and endorsing open science practices.
At the AGU annual meeting, I presented this study in a session on landslide hazard and risk analysis. The session included presentations from many other students, professors and scientists across the world, which helped me learn about the current developments in the domain of landslide hazard modeling and gain a perspective of where my research stands on a global platform. I also had some productive discussions with scientists from NASA about potential collaborations and internship opportunities that I would explore in near future. To my belief, all these experiences will greatly help me broaden my research horizons and shape my future research goals.