Josh Gray
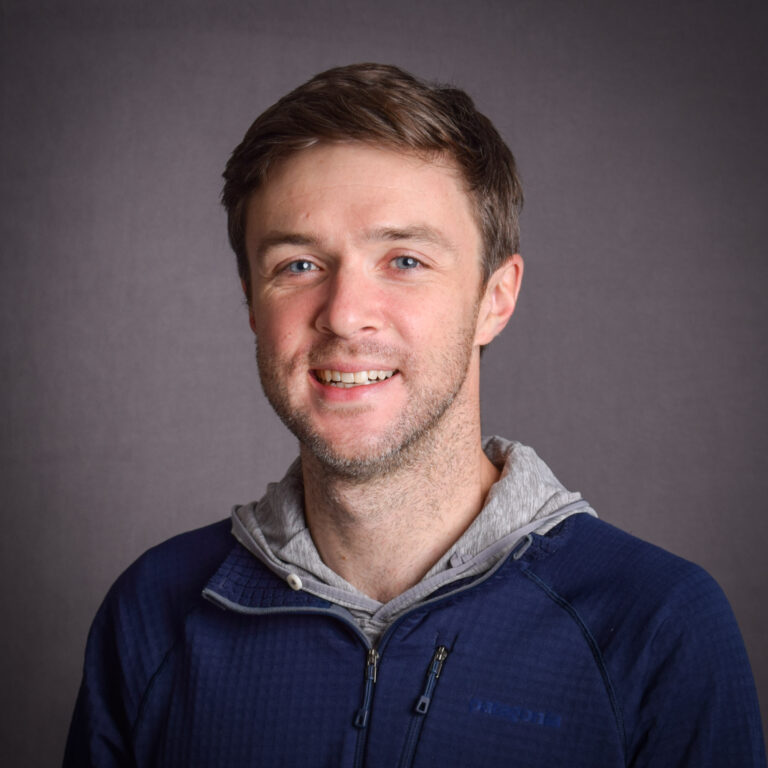
Grants
We will be using the long-term land surface phenology products we've generated to explore the climatological drivers of phenological change, trends in land surface phenology, and carbon/water consequences of these changes
This proposed SARI synthesis project for South Asia is focused on understanding LCLUC patterns and processes related to agricultural landscapes of smallholder tree-based systems (also known as trees outside of forests, TOF) and their potential as natural climate solutions. The synthesis shall provide an observation-based evaluation of the degree to which these landscapes are increasing cover and biomass, and contribute to a greatly improved understanding of the drivers of tree cover change in agricultural landscape. The outcome of the synthesis shall be a quantitative assessment of the importance of these landscapes as sinks for carbon (mitigation), both now and in the future, along with a drivers-based understanding of appropriate measures for interventions with high social and economic benefits (adaptation). We shall also evaluate their importance compared to forests landscapes both inside and outside of the national recorded forest area (RFA). Working primarily in India but expanding our analyses to the broader region, this project brings together the PIs of every current LCLUC project in South Asia, together with a cadre of the best regional scientists and organizations, as the core of a SARI South Asia LCLUC Synthesis Consortium (SARI-SAS). This Consortium will also engage past PIs from the NASA LCLUC Program working in the region. The SARI-SAS Consortium involves six US universities: Michigan State University, Columbia University, University of Minnesota, Virginia Tech, University of Florida and North Carolina State University.
Urbanization is known to have direct impacts on plant phenology. Understanding these effects is important to biodiversity dynamics, ecosystem structure, carbon cycles, and human health. Temperature increases from the Urban Heat Island (UHI) effect are thought to be the main driver of plant phenological changes around cities. However, trends in plants������������������ start of growing season (SOS) dates around urban areas, compared to their surrounding countryside, have diverged across the globe: some advance, and some delay. Divergent SOS trends have been observed in field measurements as well as satellite remotely sensed terrestrial vegetation seasonality������������������land surface phenology (LSP). However, the reasons for this phenomenon remain unclear. We hypothesize that divergent SOS trends can be explained by the interaction between UHI-induced seasonal temperature changes and variable plant chilling requirements������������������the need of plants to be exposed to sufficiently low temperatures to release dormancy in spring. This project responds directly to NASA������������������s Carbon Cycle and Ecosystems program questions: how do ecosystems, land cover and biogeochemical cycles respond to and affect global environmental change? And: What are the consequences of land cover and land-use change for human societies and the sustainability of ecosystems? Also, it is well-aligned with the broader NASA Earth Science Division goal of detecting and predicting changes in Earth������������������s ecosystems and biogeochemical cycles, including land cover, biodiversity, and the global carbon cycle. Moreover, we expect this work will make contributions to the remote sensing, phenology, and global change science communities by providing: 1) an improved understanding of how temperature controls plant phenology in urban areas; 2) a long-term 30 m spatial resolution LSP dataset for large cities and its retrieving model, which can be generally applied to other urban or natural regions.
This proposal responds to ROSES2019 A.33 (Earth Science Research from Operational Geostationary Satellite Systems), focusing on the development of high-spatial-and-temporal environmental data products from geostationary satellite observations. Specifically, we propose to develop and implement an operational near real-time land surface phenology (NRT-LSP) product in support of agriculture and forest management. The NRT-LSP product has the capability of providing near real-time monitoring and short-term prediction of vegetation phenology development. In this proposal, ����������������near real-time monitoring��������������� is referred to as the detection of phenological events occurring before the date of latest available satellite observation, and ����������������short-term prediction��������������� as the estimation of phenological events within next 10 days (10 days ahead) during a vegetation growing season. This product will be implemented at 500 m pixels, biweekly, and with a latency less than one week. LSP reflects an interaction between plant������������������s life-cycle events and environmental variables and is one of the simplest and most effective indicators of environmental change. Documenting LSP change supports efforts to reconstruct the historical environmental data record and to make predictions about biological responses to future environmental scenarios. To date, various LSP products have been produced annually and with latency greater than a year using polar-orbiting satellite datasets including AVHRR, MODIS, and VIIRS during the past several decades. Because of frequent cloud contaminations, it is infeasible to utilize these same techniques to detect LPS in near real time, or to forecast near-term phenological development at regional or global scales. Existing LSP data have been invaluable for retrospective analyses, but there would be great social, cultural, and economic value in a reduced latency LSP product. Such an NRT-LSP product would be particularly important in assisting farmers and agricultural agencies for predicting the optimum timing of cultivation practices, monitoring crop growth, and estimating crop yield; foresters for detecting disturbances related to forest pests, disease outbreaks, and species invasion; weather modelers for estimating surface energy balance in the numerical weather prediction models; and tourists for seeing spring wildflowers and fall foliage colors. Indeed, there is an urgent and substantial need to produce a near real-time LSP product. The high frequency of diurnal observations from geostationary satellites (GOES-16/17, Himawari-8/9, GEO-KOMPSAT-2A, and the upcoming EUMETSAT Meteosat Third Generation) maximizes the number of cloud-free views for creating a daily cloud-free trajectory of vegetation greenness. This offers a unique opportunity to generate an NRT-LSP product for quantifying the timing of key phenological transitions and vegetation growth conditions. This proposal describes a project that will operationally produce an NRT-LSP product at 500 m spatial resolution, with a biweekly update over the ABI full disk (North American and South America). The specific goals of this project are: ��������������� Establish climatological greenness trajectories from historical MODIS satellite data ��������������� Calculate daily angularly-corrected vegetation greenness from diurnal ABI observations ��������������� Generate synthetic time series of daily vegetation greenness by fusing ABI and VIIRS data to improve spatiotemporal resolution ��������������� Simulate potential trajectories of LSP development from available ABI-VIIRS synthetic time series and MODIS climatological vegetation greenness trajectories ��������������� Produce phenometrics (phenological timing and greenness magnitude) during a vegetation growing season in near real time and 10 days ahead ��������������� Validate the proposed phenometrics using in-situ and Phenocam observations ��������������� Evaluate the the NRT-LSP for monitoring crop and forest growth and invasive species
More than a third of crop yields are currently lost due to abiotic and biotic stressors such as drought, pests, and disease. These stressors are expected to worsen in a warmer, drier future, resulting in crop yields further declining ~25%; however, breeding is only expected to rescue 7-15% of that loss [1]. The plant microbiome is a new avenue of plant management that may help fill this gap. All plants have fungi living inside their leaves (����������������foliar fungal endophytes���������������). This is an ancient and intimate relationship in which the fungi affect plant physiology, biotic and abiotic stress tolerance, and productivity. For example, some foliar fungi prevent or delay onset of major yield-limiting diseases caused by pathogens such as Fusarium head blight [2]. Foliar endophytes also reduce plant water loss by up to half and delay wilting by several weeks [3, 4]. Endophyte effects on plants occur via diverse genes and metabolites, including genes involved in stress responses and plant defense [5]. Genes and metabolites also predict how interactions in fungal consortia affect host stress responses, which is important for developing field inoculations [6]. Because newly emergent leaves lack fungi, endophytes are also an attractive target for manipulation (particularly compared to soils, where competition with the existing microbial community inhibits microbial additives). We propose to address the role of endophytic ����������������mycobiomes��������������� in stress tolerance of five North Carolina food, fiber, and fuel crops (corn, hemp, soybean, switchgrass, wheat), and to develop tools that can push this field beyond its current limits. Our major objectives (Fig. 1) are to: 1. Identify key microbiome scales to optimally manage endophytes 2. Determine fungal mechanisms via greenhouse tests, modeling, and genetic engineering 3. Build tools for field detection of endophytes 4. Understand the regulatory environment and engage diverse stakeholders Results of these objectives will allow us to make significant progress in both understanding the basic biology of plant-fungal interactions and managing those interactions in real-world settings. Our extension efforts will also bring these ideas to the broader community. Finally, we will also be well positioned to pursue several future research endeavors supported by federal granting agencies.
Agricultural transformations have increased food production five-fold in South Asia, but that progress has not been realized in the Eastern Indo-Gangetic Plains (EGP), a region spanning India, Nepal, and Bangladesh. Meeting future food demand while coping with climate change will require substantial adaptation by EGP farmers. But we know little about the nature or outcomes of agricultural adaptations by EGP farmers, and even less about future possibilities. Our proposed research will answer the question: What is the adaptive potential of smallholder agriculture in the EGP? Our central hypotheses are: 1) Smallholder farmers have already adapted to a changing climate by planting earlier, adopting faster maturing varieties, and switching crop types. 2) These adaptive practices have mitigated the effect of climate change on crop yields. And, 3) additional transformations will further increase crop yields and resilience but socioeconomic barriers prevent widespread adoption. We will test these hypotheses by combining innovative remote sensing analyses, statistical and biophysical crop yield modeling, in-region field data collection, and causal analyses of fused household survey and remote sensing datasets. We will quantify contemporary cropping patterns and practices, and the extent and spatiotemporal variation of adaptive strategy adoption with remotely sensed assets and available ground and administrative data from regional partners. The effect of future climate change under various scenarios of agricultural adaptation will be quantified using climate projections and yield models. These analyses are integrated with a household survey and choice experiments that will reveal farmer������������������s attitudes towards climate change, adaptive agricultural practices, and the barriers to further transformation. Our effort will produce annual cadence, finely resolved maps of crop types, including the characterization of multicropping rotations, the timing and duration of critical crop growth stages, and changes in these variables over the period 2001-present. No existing products map these variables at the scale of individual smallholder fields, and for the time period and temporal cadence necessary to evaluate the adaptive potential of the EGP. We will create these products using a newly developed approach to data fusion capable of assimilating a wide variety of heterogeneous satellite imagery, including newly available high resolution commercial assets. We will use phenology algorithms to extract the timing of growth stages, and emerging approaches to classification that use a Bayesian framework to assimilate existing heterogeneous crop type maps and ancillary data. Statistical and biophysical crop yield models will be fit, driven by historical weather and downscaled climate projections, and used to quantify the climate mitigating effects of adaptive practices. Our household surveys and analysis of map products will guide the design of realistic future scenarios of agricultural adaptation. By characterizing and quantifying the adaptive potential of smallholder agriculture in the EGP, our study will support decision makers, regional food and water security, efforts to alleviate rural poverty, and the adoption of feasible climate adaptive strategies. Our project will further develop and apply innovative remote sensing methodologies such as data fusion and classification approaches, and will thus be useful to the broader remote sensing science community. Additionally, because the goals of our project are well-aligned with those of several large initiatives like SARIN, CIMMYT, and GEOGLAM, we expect our results to find a broad audience with the means and impetus to ensure they support on-the-ground change, and ultimately, a more sustainable and resilient food future for the EGP.
We propose to build a system producing near-realtime SMART datacubes for broad area search to identify candidate areas of change. The system can, then, isolate these areas of interest and create an enhanced SMART datacube around them ��� maximizing spatial resolution and temporal completeness ��� as needed. This architecture can be used for forensic analysis and change monitoring of current events with the possibility to tip-and-cue complementary future imagery products (e.g. through new tasking) to build such enhanced SMART datacube in operational settings. From this proposed research we envision an outcome that shall consist of a product that analyzes and screens over a broad area and is used to task and/or collect as much high resolution commercial imagery available or complementary imagery to do change detection, attribution and characterization (DAC) that can generate alerts for current events, as well as produce comprehensive forensic reports from completed activities or events. The broad area search will identify ���hotspots��� and will provide context on what data sources should be used to build the enhanced SMART datacube. We will conduct research to determine the optimal configuration of both near-realtime and enhanced SMART datacubes (e.g. optimal GSD for near-realtime and enhanced cubes, data sources to satisfy temporal requirements, number of spectral bands needed to detect change in large areas, etc.). Our data fusion framework shall support fusion of very diverse data sources (e.g. WorldView-1,2,3, Sentinel-2, Landsat, and other commercial providers) to conduct these experiments, as well as supporting consumption of new tasking.
As one of the main drivers of biodiversity loss, deforestation is a major issue in Myanmar and has been increasing since the democratization of the country in the 1990s. Though regulations against deforestation are in place, enforcement is often unreliable due to the latency and coarseness of available forest loss data. However, recent advances in remote sensing have made the ability of near real-time (NRT) monitoring possible at smaller scales. Although NRT monitoring methods have become much better in recent years, for the most part they remain methods that create daily deforestation (or ����������������change���������������) alerts. To our knowledge, the best methods are consistently accurate to deforested areas of 6 ha in size. This is helpful for identifying larger instances of clear-cutting, for example, but small-scale methods are necessary for smaller forested areas that primarily endure selective logging. I therefore propose to utilize these developments, combined with advanced Bayesian statistical methods and cloud-based high-power computing (e.g. Google Earth Engine), to create a continuously ground-validated, reduced-latency deforestation monitoring system for local forest managers. Given how quickly deforestation can occur at small scales before being noticed with current methods, and the rapidity with which satellite data is available, this research represents a logical step forward by building off existing work such as change detection analyses and Bayesian statistics. Also to our knowledge, this will be the first study to combine satellite data with Bayesian statistical analysis for the purpose of moving toward NRT monitoring. Successful implementation will majorly improve conservation efforts in Myanmar and subsequently forests around the world.
North Carolina State University would be pleased to confirm our commitment to serve as a subawardee to RTI in support of the competition for the Millennium Challenge Corporation cooperative agreement application in response to funding opportunity 95332418N0002, PARTNERSHIPS with MCC Program, for a proposed period of performance anticipated to be for a period of up to 30 months. Our organization will be supporting this team by providing general oversight of the project, ensuring project advancement, and providing supervision of the Research Practitioner and Graduate Student.
We propose to build a system producing near-realtime SMART datacubes for broad area search to identify candidate areas of change. The system can, then, isolate these areas of interest and create an enhanced SMART datacube around them ������������������ maximizing spatial resolution and temporal completeness ������������������ as needed. This architecture can be used for forensic analysis and change monitoring of current events with the possibility to tip-and-cue complementary future imagery products (e.g. through new tasking) to build such enhanced SMART datacube in operational settings. From this proposed research we envision an outcome that shall consist of a product that analyzes and screens over a broad area and is used to task and/or collect as much high resolution commercial imagery available or complementary imagery to do change detection, attribution and characterization (DAC) that can generate alerts for current events, as well as produce comprehensive forensic reports from completed activities or events. The broad area search will identify ���������������hotspots������������������ and will provide context on what data sources should be used to build the enhanced SMART datacube. We will conduct research to determine the optimal configuration of both near-realtime and enhanced SMART datacubes (e.g. optimal GSD for near-realtime and enhanced cubes, data sources to satisfy temporal requirements, number of spectral bands needed to detect change in large areas, etc.). Our data fusion framework shall support fusion of very diverse data sources (e.g. WorldView-1,2,3, Sentinel-2, Landsat, and other commercial providers) to conduct these experiments, as well as supporting consumption of new tasking.